You signed in with another tab or window. Reload to refresh your session.You signed out in another tab or window. Reload to refresh your session.You switched accounts on another tab or window. Reload to refresh your session.Dismiss alert
파이토치 같은 경우 각 GPU마다 같은 프로그램을 띄워서 필요할 때 각 프로그램이 collective operation을 수행하는 방식.
TF v1 같은 경우는 하나의 프로그램에서 computation graph를 쪼개 각 worker들에 대해 나눠주는 방식이었음.
DEV == device (TPU)
multi controller가 기본적으로 더 효율적이긴 하지만 유연하지는 않음.
파이프라이닝을 한다거나 모델이 sparse한 task들로 구성되어 있어 서로 다른 worker에서 서로 다른 연산을 수행하는 것이 자연스럽다면 효율성이 떨어짐
Pathways는 single controller 모델의 유연성에 multi controller 모델의 효율성을 결합할 수 있는가를 태클하기 위한 제안.
SPMD == Single Process Multi Data
MPMD == Multi Process Multi Data
다른 말로 하면, 기존 방식에서 벗어나 MPMD 를 support 하는 것이 목표.
이를 위해서는 Gang-scheduling 이 반드시 필요함. TPU 가 single-threaded 하게 동작하기 때문.
실제 jax 에서 사용예시를 보면 굉장히 간단함.
함수별로 device 를 할당해주고, 돌려주면 끝.
이젠 진짜 JAX 를 써봐야 하는건가...
위 알고리즘 예시를 보면, (A-B) 연산 후 return 값과 (A-C-B) 연산 후 return 값 2개가 있다.
이를 DAG 로 나타내면 아래 그림의 가장 왼쪽 그림이 된다.
그 이후, ResourceManager 가 각 연산에 필요한 island를 할당하고 (TPU 자원 할당)
Gang schduler 가 이를 최대한 효율적인 방식으로 돌려주는 역할을 수행한다.
dispatch 는 scheduler 가 ready state 의 process 를 runnning state 로 바꾸는 과정이다.
이 작업은 흔히 dispatcher 가 수행한다.
dispatcher 를 잘 설계하면, scheduling 의 성능을 끌어올릴 수 있다.
아래 그림의 (a) 는 단순한 sequential disaptch 를 보여준다.
노란작업, 초록작업, 파란 작업이 순서대로 수행된다.
하지만, send, recv 과정의 시간 소요를 줄이는 게 좋기 때문에, (b) 와 같이 parallel dispatch 를 하는 편이 효과적이다.
sequential 하게 처리하는 부분은, 작업이 끝났다고 알리는 "send" 만 사용하면 된다.
이를 통해 2048 TPU 에서서 100% device util을 뽑아낼 수 있었다고 한다.
The text was updated successfully, but these errors were encountered:
paper
TPU들에 대해 어떻게 분산 처리를 할 것인가
파이토치 같은 경우 각 GPU마다 같은 프로그램을 띄워서 필요할 때 각 프로그램이 collective operation을 수행하는 방식.

TF v1 같은 경우는 하나의 프로그램에서 computation graph를 쪼개 각 worker들에 대해 나눠주는 방식이었음.
DEV == device (TPU)
multi controller가 기본적으로 더 효율적이긴 하지만 유연하지는 않음.
파이프라이닝을 한다거나 모델이 sparse한 task들로 구성되어 있어 서로 다른 worker에서 서로 다른 연산을 수행하는 것이 자연스럽다면 효율성이 떨어짐
Pathways는 single controller 모델의 유연성에 multi controller 모델의 효율성을 결합할 수 있는가를 태클하기 위한 제안.
SPMD == Single Process Multi Data
MPMD == Multi Process Multi Data
다른 말로 하면, 기존 방식에서 벗어나 MPMD 를 support 하는 것이 목표.
이를 위해서는 Gang-scheduling 이 반드시 필요함. TPU 가 single-threaded 하게 동작하기 때문.
실제 jax 에서 사용예시를 보면 굉장히 간단함.
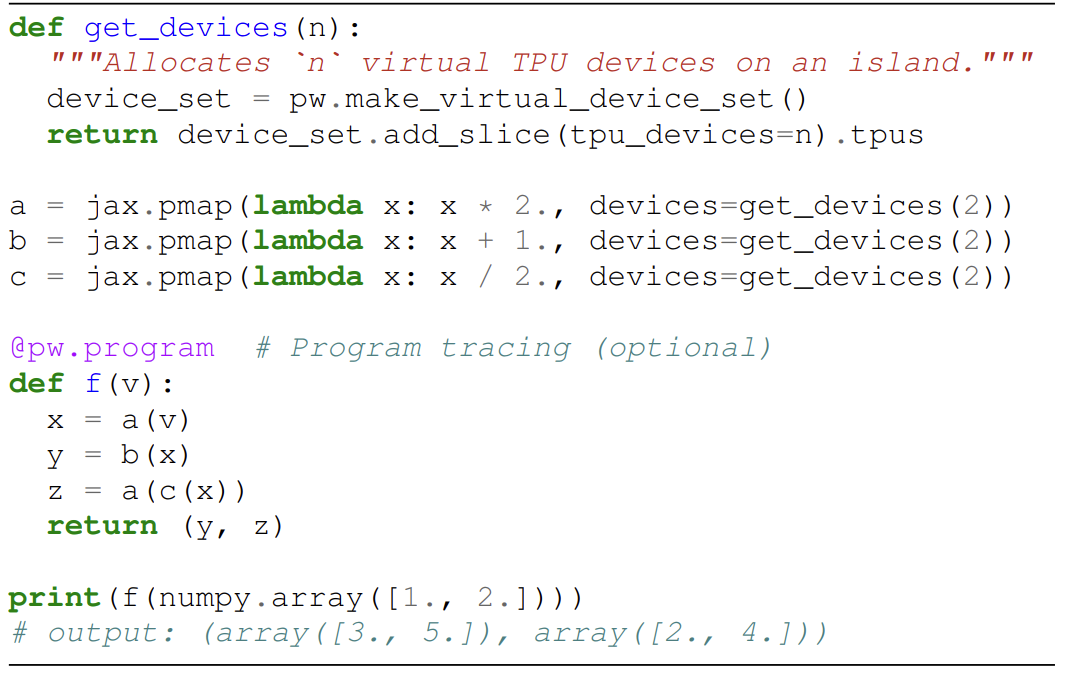
함수별로 device 를 할당해주고, 돌려주면 끝.
이젠 진짜 JAX 를 써봐야 하는건가...위 알고리즘 예시를 보면, (A-B) 연산 후 return 값과 (A-C-B) 연산 후 return 값 2개가 있다.
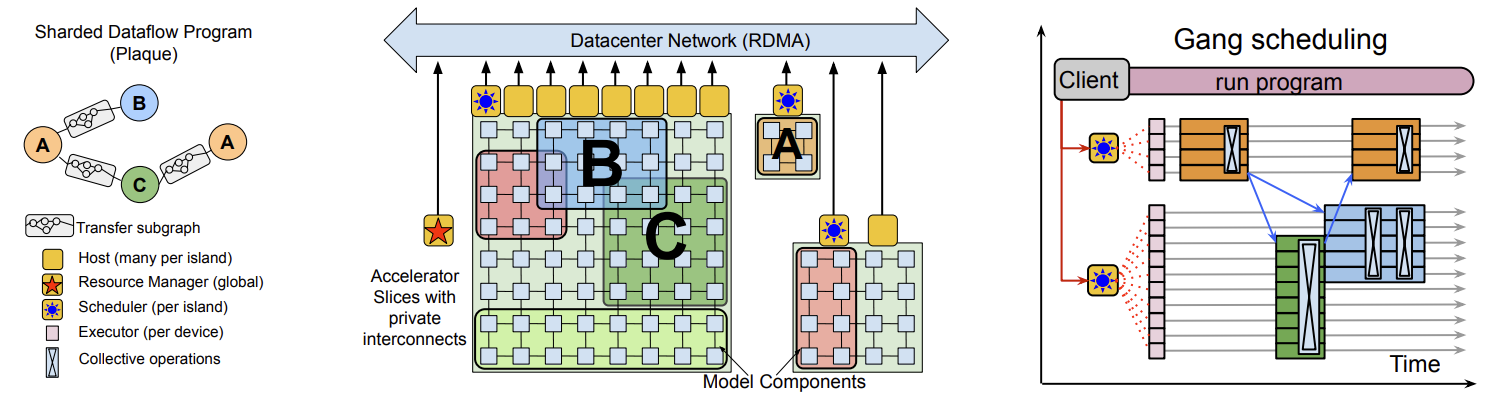
이를 DAG 로 나타내면 아래 그림의 가장 왼쪽 그림이 된다.
그 이후, ResourceManager 가 각 연산에 필요한 island를 할당하고 (TPU 자원 할당)
Gang schduler 가 이를 최대한 효율적인 방식으로 돌려주는 역할을 수행한다.
dispatch 는 scheduler 가 ready state 의 process 를 runnning state 로 바꾸는 과정이다.
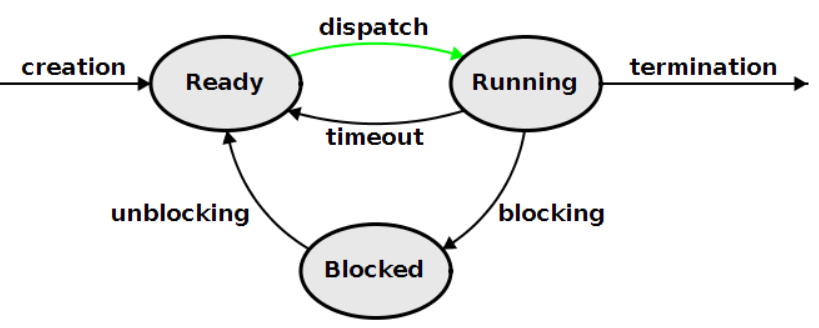
이 작업은 흔히 dispatcher 가 수행한다.
dispatcher 를 잘 설계하면, scheduling 의 성능을 끌어올릴 수 있다.
아래 그림의 (a) 는 단순한 sequential disaptch 를 보여준다.
노란작업, 초록작업, 파란 작업이 순서대로 수행된다.
하지만, send, recv 과정의 시간 소요를 줄이는 게 좋기 때문에, (b) 와 같이 parallel dispatch 를 하는 편이 효과적이다.
sequential 하게 처리하는 부분은, 작업이 끝났다고 알리는 "send" 만 사용하면 된다.
이를 통해 2048 TPU 에서서 100% device util을 뽑아낼 수 있었다고 한다.
The text was updated successfully, but these errors were encountered: