You signed in with another tab or window. Reload to refresh your session.You signed out in another tab or window. Reload to refresh your session.You switched accounts on another tab or window. Reload to refresh your session.Dismiss alert
6144 TPU v4 chip 을 이용해 540B GPT-like 모델 학습
Pathways system 사용 (#115)
paper 에서 주로 볼 내용
Efficient Scaling : Pathways system 을 어떻게 활용하였는가
Continued improvements from scaling : 몇 가지 테크닉들을 썼더니, SOTA 를 찍더라. LLM을 정복하기 위해 우리의 갈 길이 멀다.
Breakthrough capabilities: 기존에 잘 안되던 task 들에서도 잘 된다. BIG-bench 에서 꽤 큰 성능향상
Discontinuous improvements : 8B, 62B, 540B 로 점차 모델 크기를 늘려갔지만, power law 이상으로 540B에서 갑자기 성능이 뛰더라.
Multilingual understanding : non-English data 에서도 잘 동작하더라 (사용한 data 의 22% 정도만 non-English 인데도, SOTA)
Bias and toxicity : SOTA
Model Architecture
개인적으로 여기에 관심이 많이 갔다
SwiGLU activation
Swish(xW) · xV 형태의 activation 이다. GLU variants improve transformer 에서 제안되었고, 해당 논문에서는 ReLU variant model + larger dimension 등. compute-equivalent 한 setting 들에서 비교해 보니, 더 좋았다고 주장한다.
이 방식은 15% 정도 training 속도가 빠르다.
8B scale 에서 학습결과가 약간 안 좋았고, 62B scale 에서는 거의 차이가 없었다고 한다.
저자들은 540B 학습 용도로 적합하다 판단하고 사용했다.
Multi-Query Attention
Fast transformer decoding: One write-head is all you need 에서 제안했었다.
k == attnetion head 개수
h == attention head dimension
원래 attention 은 query key value 각각을 [k, h] 로 projection 시켰는데,
여기서는 key/value projection 을 head 별로 모두 공유한다.
즉, key, value 는 [1, h] 형태인 거고 모든 head 에서 공유.
이것도 성능에는 크게 영향이 없으면서, autoregressive decoding time 은 크게 개선한다.
아무래도 standard multi-head attention으로 autoregressive decoding 을 수행하는게 accelerator 에서 효율적이지 않다 보니 어쩔 수 없다.
layernorm 이나 deense kernel 어느 곳에도 bias 를 사용하지 않았다.
이렇게 하니 학습이 안정되더라
Vocabularay
SentencePiece 에 256k token 활용. (다양한 언어 커버치게 설정)
training data 를 기반으로 학습 efficienct 가 좋은 애들로 설정.
out of vocab Unicode character 들은 UTF-8 byte 로 split 되었고, 숫자들은 individual digit token 들로 나누었다. ("123.5 → 1 2 3 . 5")
기존 많은 LM 들이 HFU (Hardware FLOPs Utilization) 을 사용해 왔다. 이론적인 FLOPs 를 재는 방식이다.
이는 기기마다 다를 수 있고, 실재 속도를 반영하지 못하기 때문에, MFU (Model FLOPs Utilization) 을 제안한다.
observed througput 을 theoretical maximum throughput 으로 나눠준 방식이다.
구글... 인프라.. 부럽다..
Training Instability
이게 참 신기했다. 540B 모델 학습하는데 20번 정도 loss 가 튀더라.
gradeient clip 을 썼는데도 튀더라.
이를 해결하기 위해 그냥 100 step 마다 checpointing 을 수행하고, spike 가 발생하기 시작하면, 200~500 batch 만큼 데이터 샘플들을 넘겨서 학습시켰다.
저자들은 이 데이터들이 "bad data" 라고 생각하지는 않는다. 같은 데이터를 통과시킬 때, 모델의 state (weight 값) 가 다르면, spike 가 안 튈 때도 있기 때문이다. very large LM 에 대한 이런 spike 연구 또한 계획중이라 한다.
resoning 에서는 여러 문제와 정답을 알려주고 마지막 문제로 정답을 예측한다.
아래 그림의 standard prompting 과 같다.
이 때, model 입력으로 넣어주는 answer 에 reasoning 하는 과정을 넣어주니까 model output 도 비슷하게 "사고" 를 하기 시작한다는 놀라운 결과.
reasoning 에서는 chain prompting 이 역시나 상당히 잘 동작한다.
Multi-lingual
상당히 잘 동작한다. 이런 figure에 korean 은 언제쯤 보일라나 🧐
Discontinuous improvements
evaluation 은 이쯤보고 Intro 에 언급되었던 Discontinuous improvements 정도만 정리하고 마치려 한다.
task 마다 다르지만, 모델이 커질 수록 power law 에 맞춰서 성능향상이 이루어 지는 것이 아니라, 540B 에서는 성능향상이 확 일어나더라.
Chinchilla (#117) 에서의 scaling law 가 정말 큰 모델에서는 다르게 동작할 수 있겠다 생각이 들었다.
The text was updated successfully, but these errors were encountered:
paper
blog
6144 TPU v4 chip 을 이용해 540B GPT-like 모델 학습
Pathways system 사용 (#115)
paper 에서 주로 볼 내용
Model Architecture
개인적으로 여기에 관심이 많이 갔다
SwiGLU activation
Swish(xW) · xV
형태의 activation 이다.GLU variants improve transformer 에서 제안되었고, 해당 논문에서는 ReLU variant model + larger dimension 등. compute-equivalent 한 setting 들에서 비교해 보니, 더 좋았다고 주장한다.
Parallel Layers
기존 transformer 는 다음과 같이 쓸 수 있다.

GPT-J-6B 에서는 다음과 같은 Parallel Layers 를 제시했다.

이 방식은 15% 정도 training 속도가 빠르다.
8B scale 에서 학습결과가 약간 안 좋았고, 62B scale 에서는 거의 차이가 없었다고 한다.
저자들은 540B 학습 용도로 적합하다 판단하고 사용했다.
Multi-Query Attention
Fast transformer decoding: One write-head is all you need 에서 제안했었다.
k == attnetion head 개수
h == attention head dimension
원래 attention 은 query key value 각각을 [k, h] 로 projection 시켰는데,
여기서는 key/value projection 을 head 별로 모두 공유한다.
즉, key, value 는 [1, h] 형태인 거고 모든 head 에서 공유.
이것도 성능에는 크게 영향이 없으면서, autoregressive decoding time 은 크게 개선한다.
아무래도 standard multi-head attention으로 autoregressive decoding 을 수행하는게 accelerator 에서 효율적이지 않다 보니 어쩔 수 없다.
RoPE Embeddings
RoFormer 에서 제안되었던 방식이다. (GPT-J-6B 에서도 사용했었다)
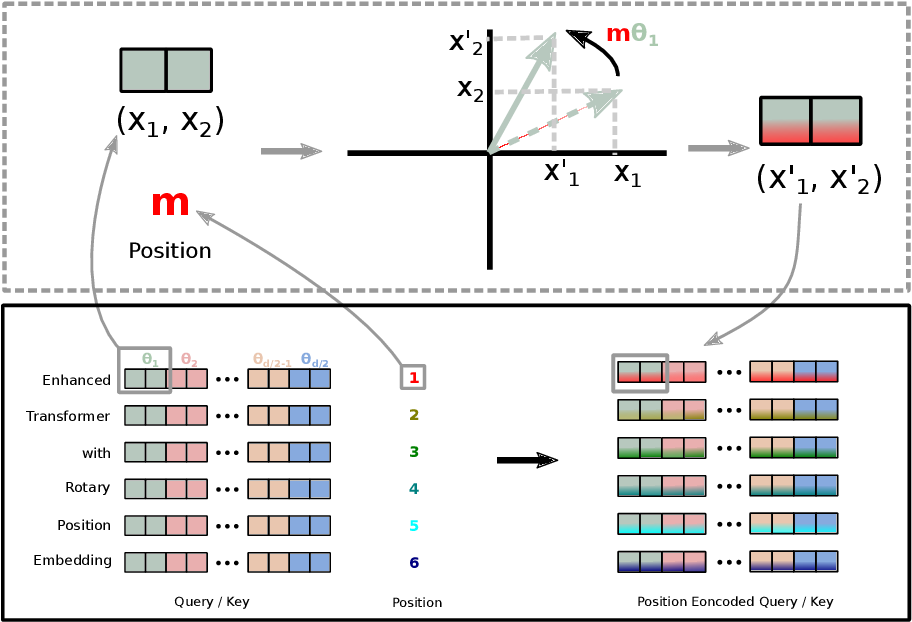
위 슬라이드 출처: https://www.slideshare.net/taeseonryu/roformer-enhanced-transformer-with-rotary-position-embedding?from_action=save
Shared Input-Output Embeddings
No Biases
layernorm 이나 deense kernel 어느 곳에도 bias 를 사용하지 않았다.
이렇게 하니 학습이 안정되더라
Vocabularay
SentencePiece 에 256k token 활용. (다양한 언어 커버치게 설정)
training data 를 기반으로 학습 efficienct 가 좋은 애들로 설정.
out of vocab Unicode character 들은 UTF-8 byte 로 split 되었고, 숫자들은 individual digit token 들로 나누었다. ("123.5 → 1 2 3 . 5")
Model Scale Hyperparameters
Training Dataset
#117 처럼 맞춰서 데이터를 더 쓰면 성능이 무조건 올라갈 것으로 보인다Training Infrastructure
2개의 TPU v4 Pod 을 사용했다. (with pathways)
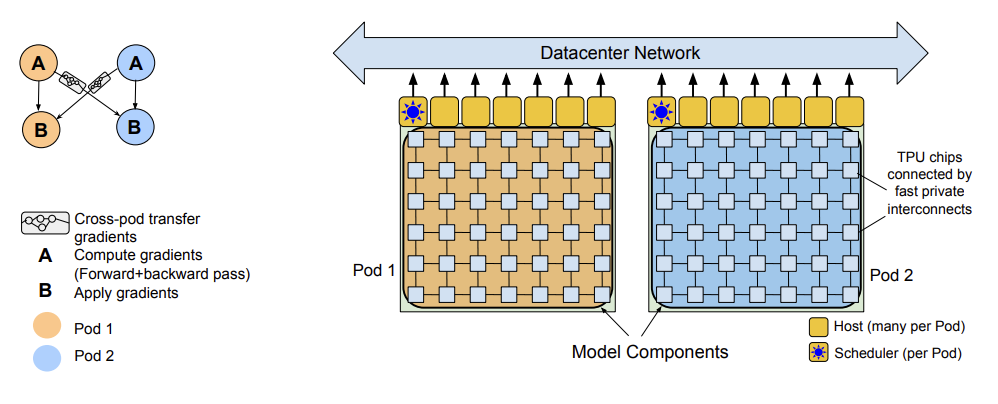
Training Efficiency
기존 많은 LM 들이 HFU (Hardware FLOPs Utilization) 을 사용해 왔다. 이론적인 FLOPs 를 재는 방식이다.
이는 기기마다 다를 수 있고, 실재 속도를 반영하지 못하기 때문에, MFU (Model FLOPs Utilization) 을 제안한다.
observed througput 을 theoretical maximum throughput 으로 나눠준 방식이다.
구글... 인프라.. 부럽다..
Training Instability
이게 참 신기했다. 540B 모델 학습하는데 20번 정도 loss 가 튀더라.
gradeient clip 을 썼는데도 튀더라.
이를 해결하기 위해 그냥 100 step 마다 checpointing 을 수행하고, spike 가 발생하기 시작하면, 200~500 batch 만큼 데이터 샘플들을 넘겨서 학습시켰다.
저자들은 이 데이터들이 "bad data" 라고 생각하지는 않는다. 같은 데이터를 통과시킬 때, 모델의 state (weight 값) 가 다르면, spike 가 안 튈 때도 있기 때문이다. very large LM 에 대한 이런 spike 연구 또한 계획중이라 한다.
Evaluation
English NLP tasks
BIG-Bench
닝겐을... 이겨버렸다..
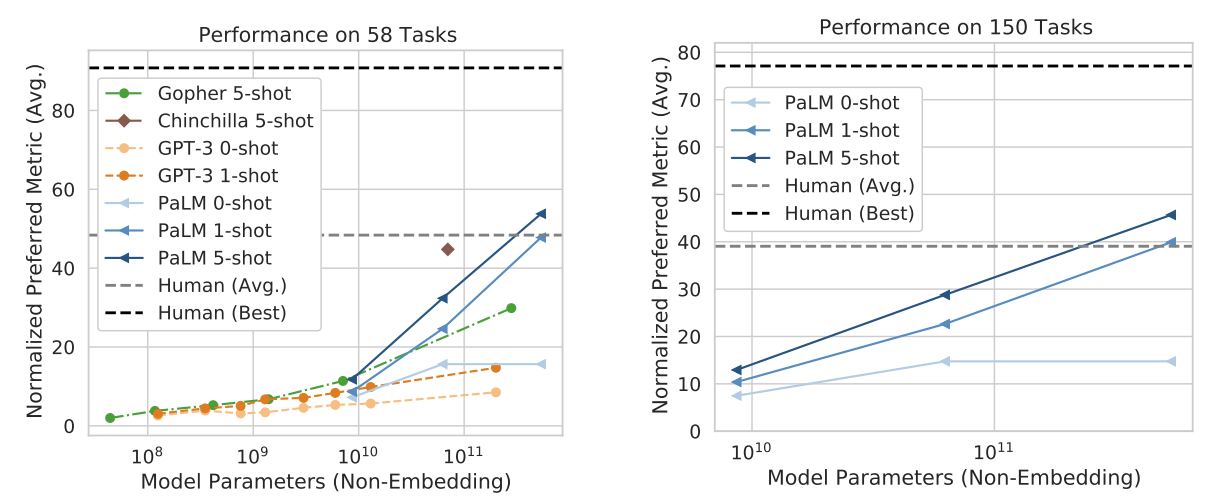
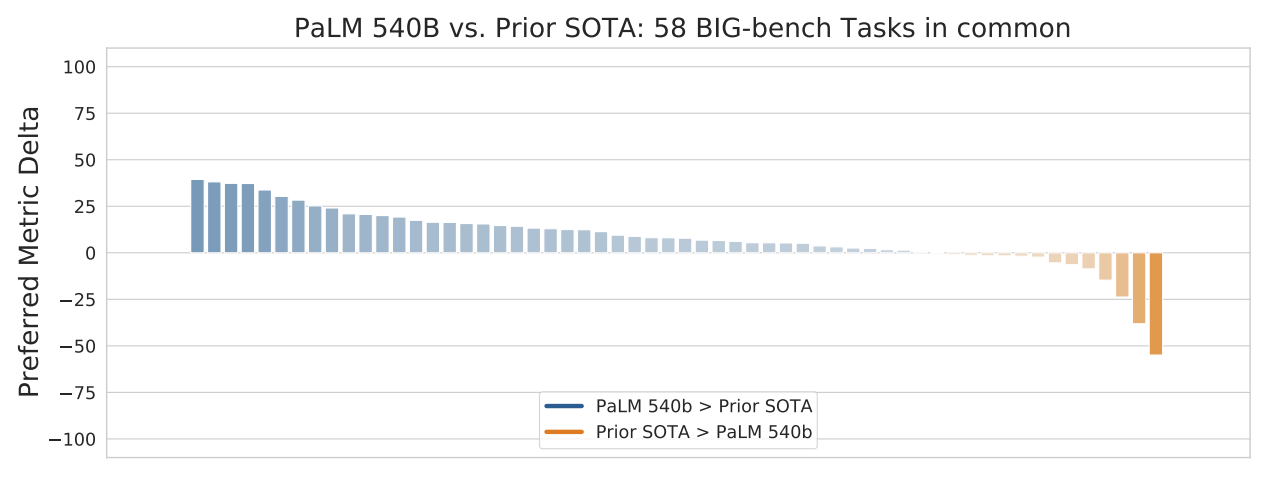
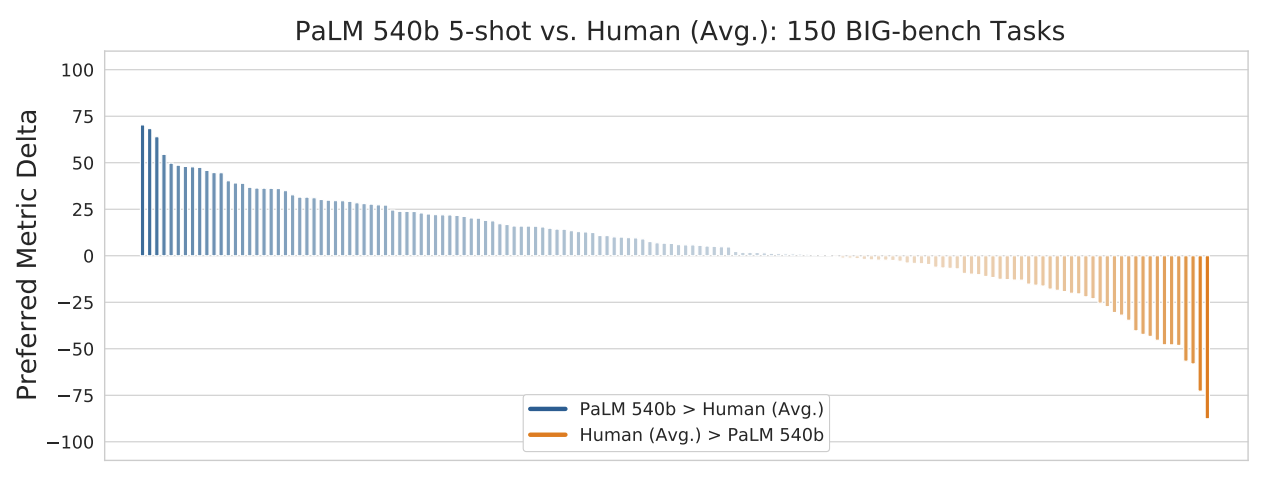
Reasoning
Chain of thought Prompting
관련 구글 논문이 또 2개가 있다.
Self-Consistency Improves Chain of Thought Reasoning in Language Models
Chain of Thought Prompting Elicits Reasoning in Large Language Models
4월 6일 기준으로 두 논문 모두 v2 로 업데이트 되었다. (PaLM 결과 포함)
resoning 에서는 여러 문제와 정답을 알려주고 마지막 문제로 정답을 예측한다.
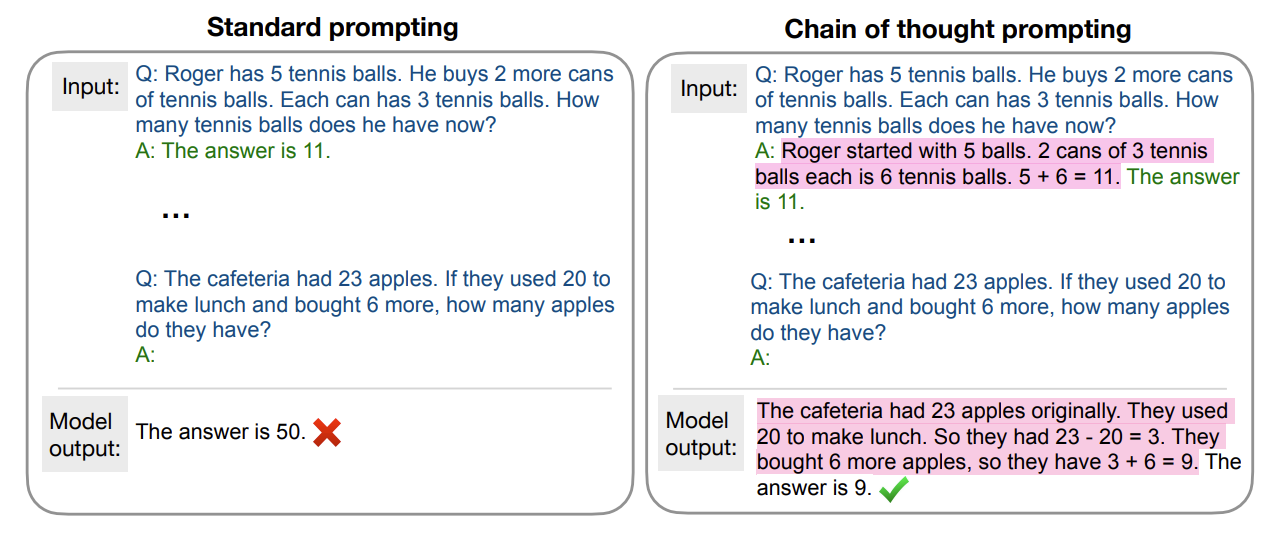
아래 그림의 standard prompting 과 같다.
이 때, model 입력으로 넣어주는 answer 에 reasoning 하는 과정을 넣어주니까 model output 도 비슷하게 "사고" 를 하기 시작한다는 놀라운 결과.
reasoning 에서는 chain prompting 이 역시나 상당히 잘 동작한다.
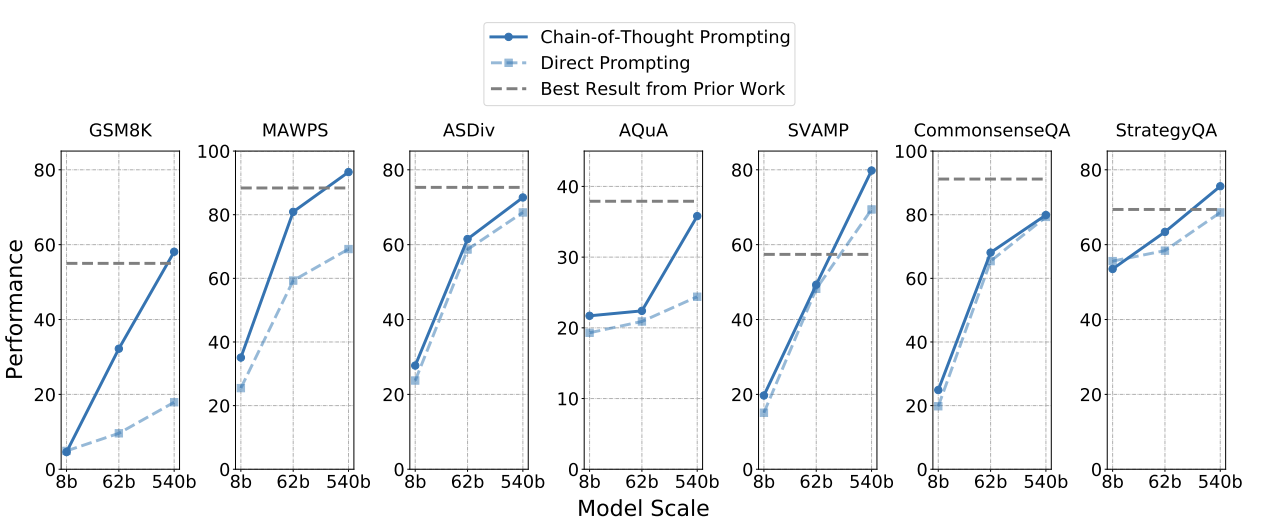
Multi-lingual
상당히 잘 동작한다.
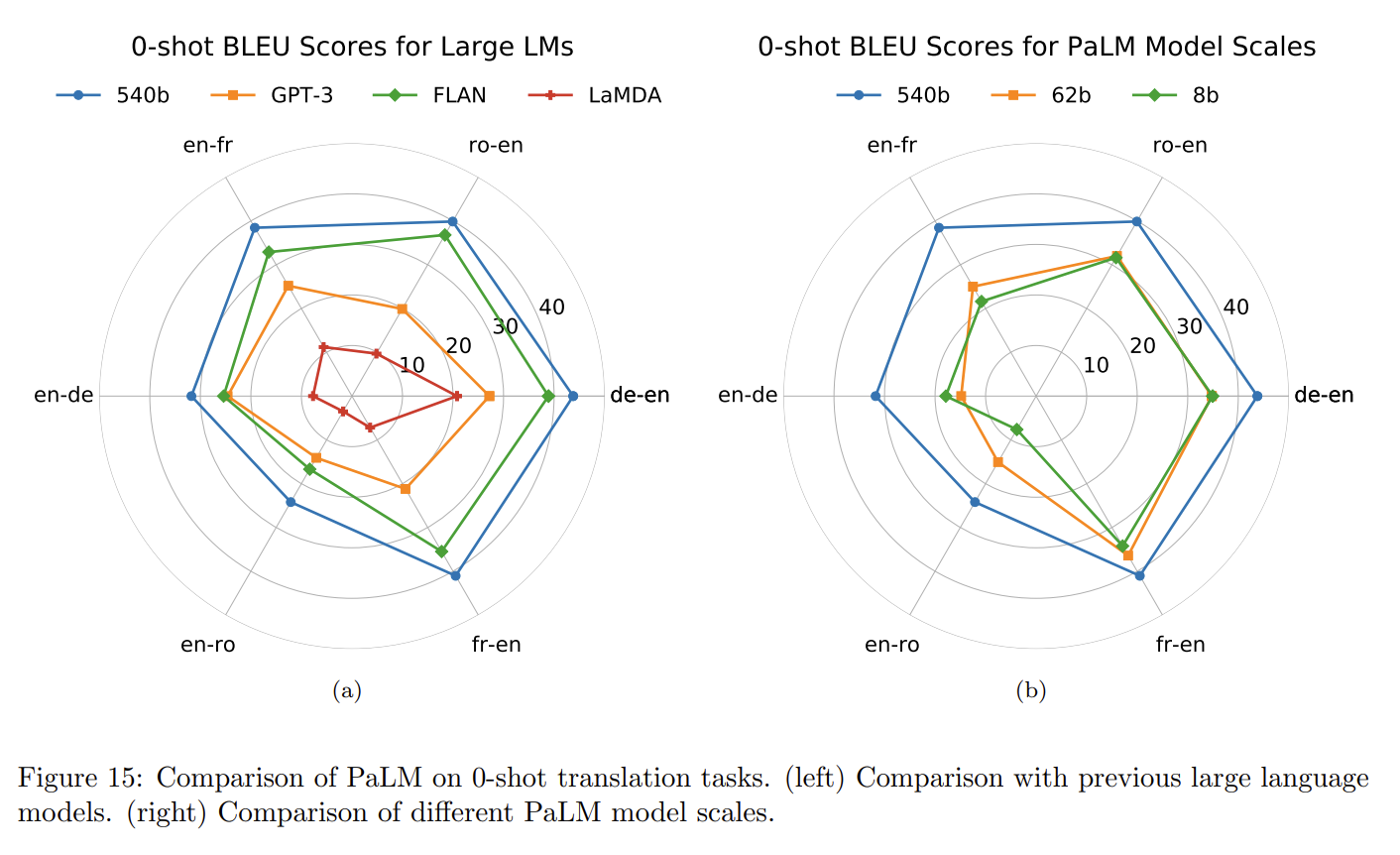
이런 figure에 korean 은 언제쯤 보일라나🧐Discontinuous improvements
evaluation 은 이쯤보고 Intro 에 언급되었던 Discontinuous improvements 정도만 정리하고 마치려 한다.
task 마다 다르지만, 모델이 커질 수록 power law 에 맞춰서 성능향상이 이루어 지는 것이 아니라, 540B 에서는 성능향상이 확 일어나더라.
Chinchilla (#117) 에서의 scaling law 가 정말 큰 모델에서는 다르게 동작할 수 있겠다 생각이 들었다.
The text was updated successfully, but these errors were encountered: