You signed in with another tab or window. Reload to refresh your session.You signed out in another tab or window. Reload to refresh your session.You switched accounts on another tab or window. Reload to refresh your session.Dismiss alert
말 그대로 attention 을 효율적으로 다루는 논문.
vit 에 적용해서 swin 보다 효율적인 것을 주장.
SepViT
간단하다. 말 그대로 depthwise 하게 attention 을 먼저 해주고, channel 별 중요도를 학습시켜서 attention 효율화를 수행한다.
제안하는 방식을 DSSA (Depthwise Seperable Self Attention) 이라 부른다.
DSSA = DSA (Depthwise Self Attn) + PWA (PointWise Attn) 이다.
win_tokens 는 학습 가능한 token 들이다.
요 녀석들을 learnable 하게 만들고 channel 별 중요도를 뽑아낸다는 개념.
Grouping
depthwise attention를 더 효율적으로 수행하기 위해서 window 를 설정하고 local 하게 살펴보게 된다.
근데, window 를 확 키워버리는게 생각보다 성능이 괜찮다. (물론 속도는 느려진다)
해당 방식을 GSA (Grouped Self Attention) 이라 한다.
아래 그림을 보면 더 이해가 잘 간다.
GSA = DSA + PWA 인건 똑같은데, window size 만 차이가 있는 것으로 보인다. (워딩이 굉장히 헷갈린다.)
paper
unofficial code - lucidrains
말 그대로 attention 을 효율적으로 다루는 논문.
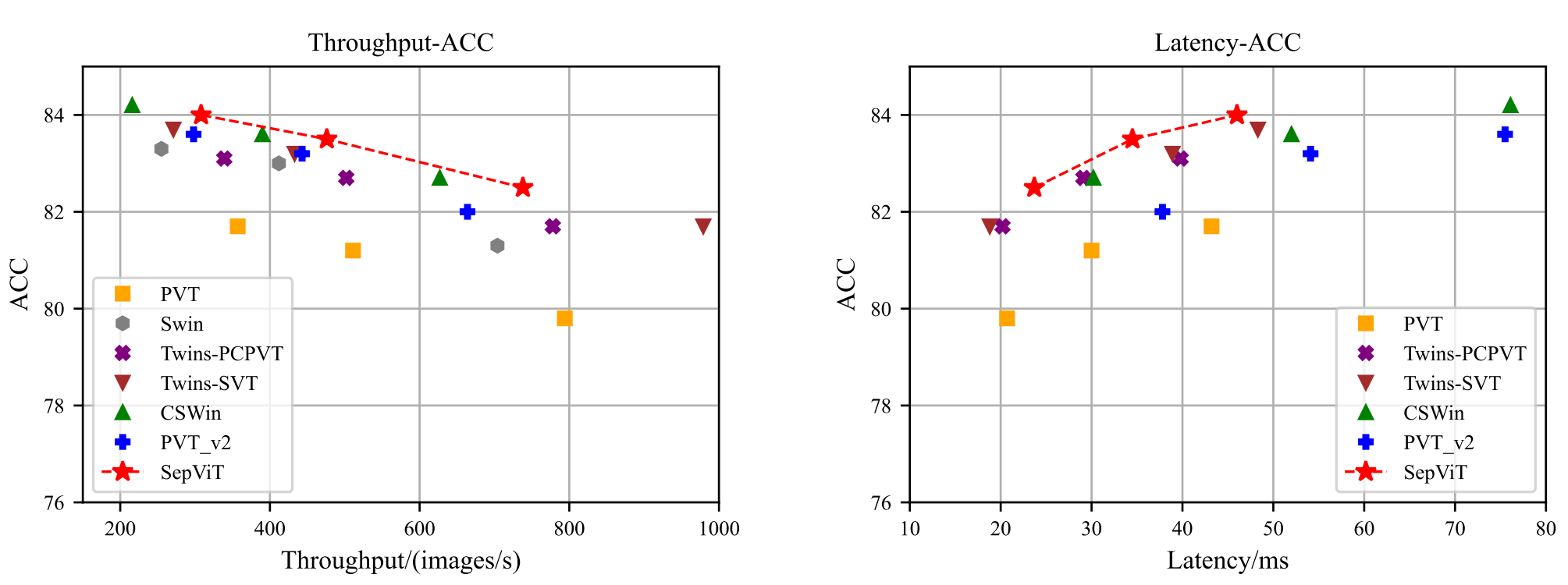
vit 에 적용해서 swin 보다 효율적인 것을 주장.
SepViT
간단하다. 말 그대로 depthwise 하게 attention 을 먼저 해주고, channel 별 중요도를 학습시켜서 attention 효율화를 수행한다.
제안하는 방식을 DSSA (Depthwise Seperable Self Attention) 이라 부른다.
DSSA = DSA (Depthwise Self Attn) + PWA (PointWise Attn) 이다.
win_tokens 는 학습 가능한 token 들이다.
요 녀석들을 learnable 하게 만들고 channel 별 중요도를 뽑아낸다는 개념.
Grouping
depthwise attention를 더 효율적으로 수행하기 위해서 window 를 설정하고 local 하게 살펴보게 된다.
근데, window 를 확 키워버리는게 생각보다 성능이 괜찮다. (물론 속도는 느려진다)
해당 방식을 GSA (Grouped Self Attention) 이라 한다.
아래 그림을 보면 더 이해가 잘 간다.
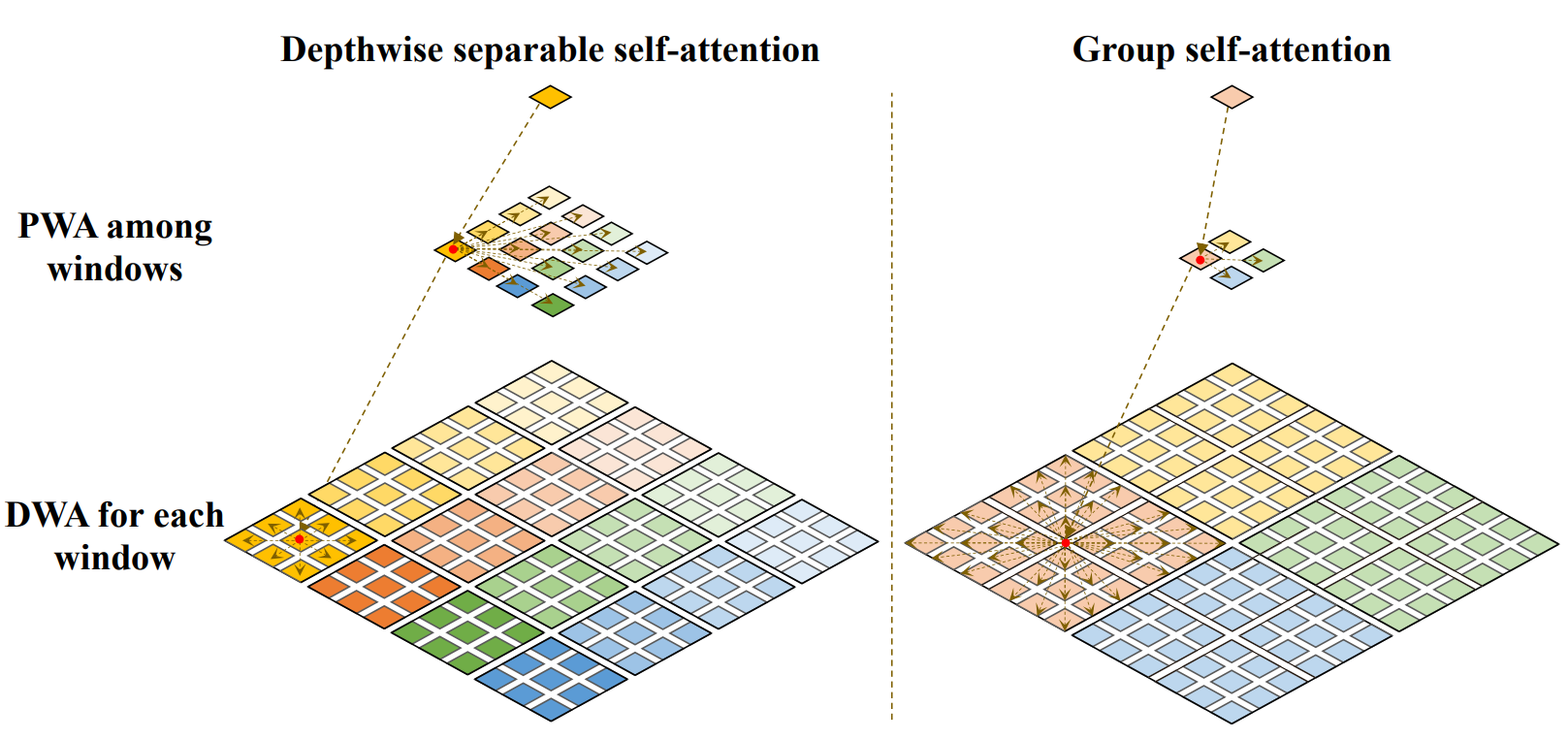
GSA = DSA + PWA 인건 똑같은데, window size 만 차이가 있는 것으로 보인다. (워딩이 굉장히 헷갈린다.)
이라고 한다.
Results
Ablations
Throughput 말고 latency도 궁금한데 흠..
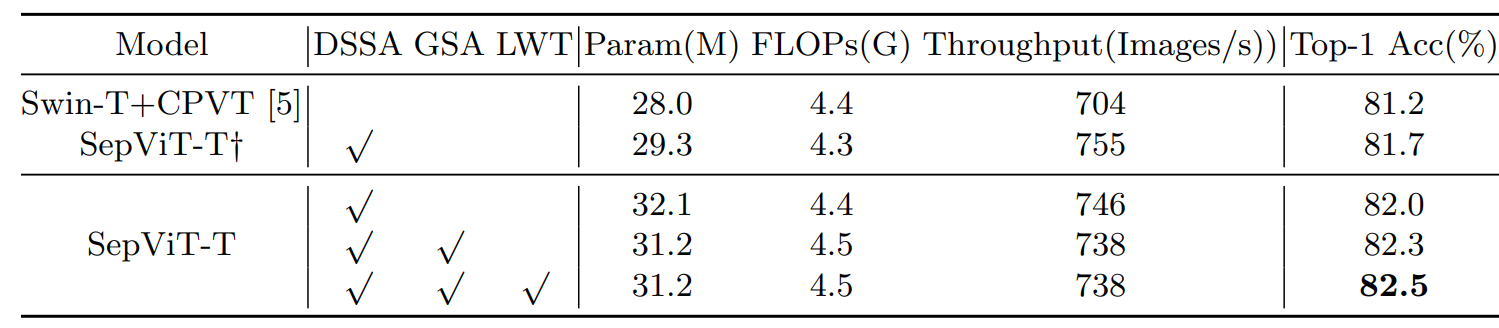
ImageNet (classification)
latency 는 T4 + TensorRT + 8 batch 로 쟀다.
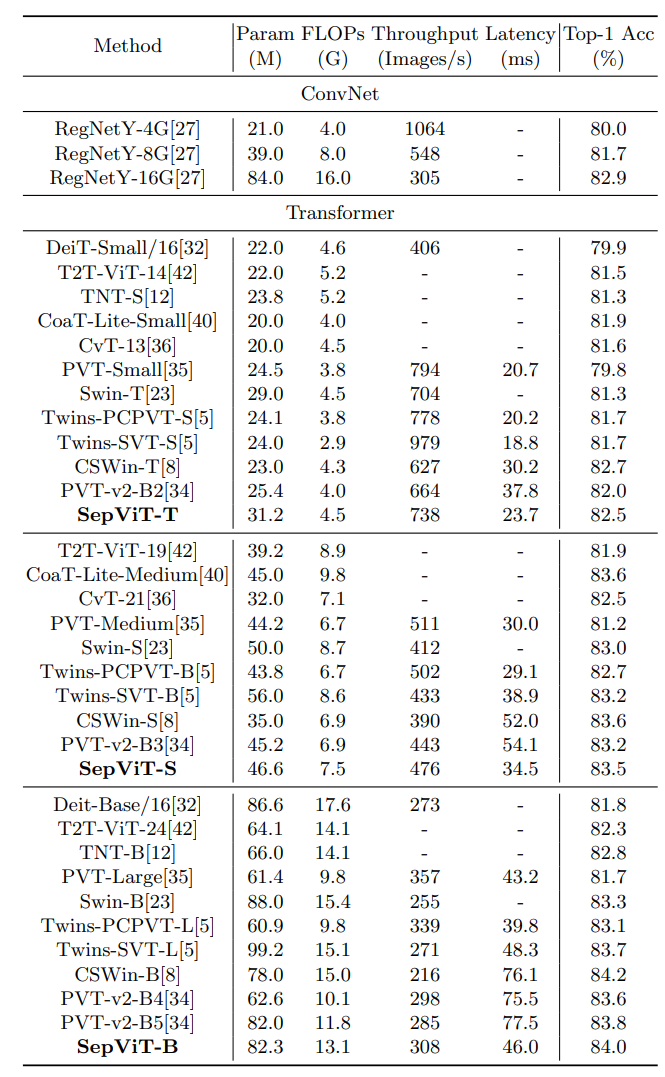
Settings
rand-m9-mstd0.5-inc1
ADE20K (semantic segmentation)
COCO (detection & instance segmentation)
The text was updated successfully, but these errors were encountered: