diff --git a/content/docs/guides/rapidocr_web/rapidocr_web_desktop.md b/content/docs/guides/rapidocr_web/rapidocr_web_desktop.md
index 9dde463f..283efbf8 100644
--- a/content/docs/guides/rapidocr_web/rapidocr_web_desktop.md
+++ b/content/docs/guides/rapidocr_web/rapidocr_web_desktop.md
@@ -20,6 +20,7 @@ categories:
#### 使用步骤
1. 下载对应的zip包
- 目前已有的zip包如下:
+

- 下载方式: [Github](https://github.com/RapidAI/RapidOCR/releases/tag/v0.1.5) | [百度网盘](https://pan.baidu.com/s/1Kfk-56I4GoKw8xMZlqUUEw?pwd=rfen) | QQ群共享(群号:755960114)
2. 解压目录如下示例
diff --git a/content/docs/guides/rapidocr_web/rapidocr_web_nuitka.md b/content/docs/guides/rapidocr_web/rapidocr_web_nuitka.md
index 7f86e88b..3dde7d4c 100644
--- a/content/docs/guides/rapidocr_web/rapidocr_web_nuitka.md
+++ b/content/docs/guides/rapidocr_web/rapidocr_web_nuitka.md
@@ -31,6 +31,7 @@ categories:
- ⚠️ `rapidocr_onnxruntime>=1.2.8`以后不用再手动修改下面代码,已经做了修改。可以跳过该步。
- 进入`rapidocr-onnxruntime`安装位置,一般在`Lib\site-packages\rapidocr_onnxruntime`或者你设置的虚拟环境下。
- 用编辑器打开`rapid_ocr_api.py`,对**39-52行**进行修改,如下图:
+
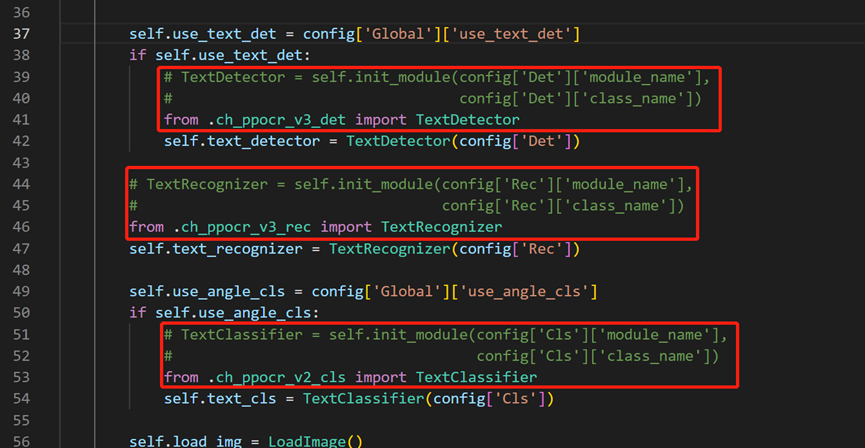
3. `nuitka`打包
```bash
@@ -38,14 +39,17 @@ categories:
nuitka --mingw64 --standalone --show-memory --show-progress --nofollow-import-to=tkinter --output-dir=out ocrweb.py
```
- 如下图所示:
+
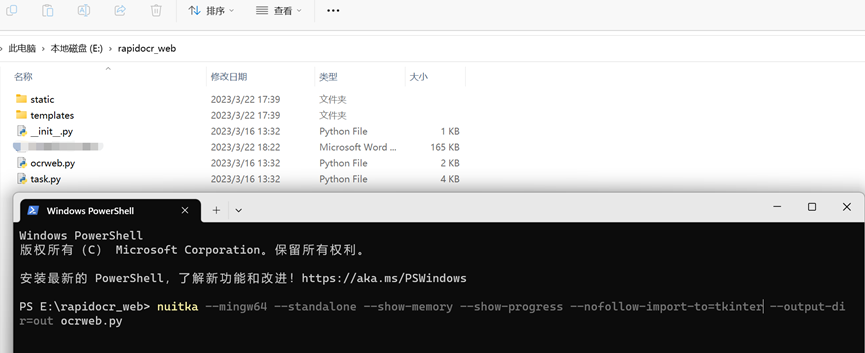
4. 拷贝静态文件
- 打包后的文件位于当前位置的`out\ocrweb.dist`目录下,需要将`web`项目和`rapidocr-onnxruntime`相关文件拷贝到此目录。
+
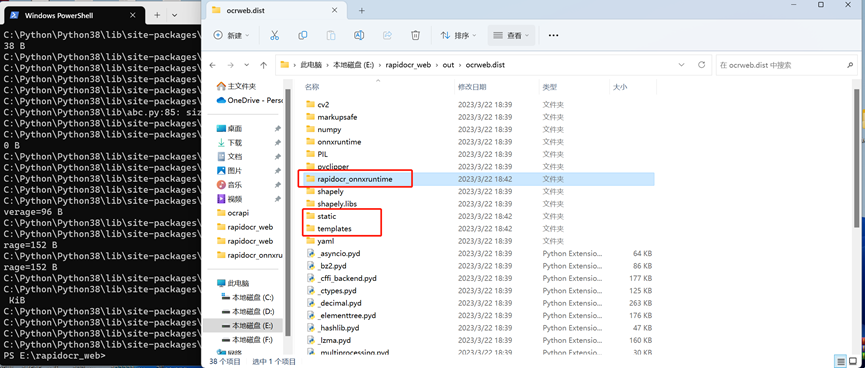
- 拷贝`rapidocr_web`目录`static`和`templates`两个文件夹全部拷贝到`out\ocrweb.dist`下
- 在`out\ocrweb.dist`创建`rapidocr_onnxruntime`文件夹,将`Lib\site-packages\rapidocr_onnxruntime`目录下的`config.yaml`和`models`文件夹拷贝到`out\ocrweb.dist\rapidocr_onnxruntime`文件夹内
5. 运行程序
- 进入`out\ocrweb.dist`,直接双击`ocrweb.exe`运行。
+
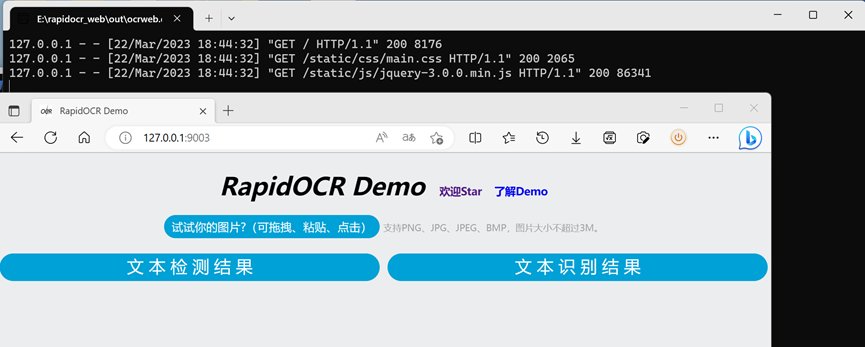
6. 打包好的exe下载:[百度网盘](https://pan.baidu.com/s/1nj_1rjuVu76drKBZDY9Bww?pwd=xnu7) | [Google Drive](https://drive.google.com/drive/folders/1okQj22XxLUptyhjKQcRU25eI8Ya693gf?usp=share_link) | [Gitee](https://gitee.com/RapidAI/RapidOCR/releases/download/v1.2.0/ocrweb.dist.rar)
diff --git a/content/docs/inference_engine/_index.md b/content/docs/inference_engine/_index.md
new file mode 100644
index 00000000..3ab12f62
--- /dev/null
+++ b/content/docs/inference_engine/_index.md
@@ -0,0 +1,10 @@
+---
+weight: 400
+title: "推理引擎相关"
+description:
+icon: menu_book
+date: 2023-09-13
+lastmod: 2023-09-13
+draft: false
+images: []
+---
diff --git a/content/docs/inference_engine/onnxruntime/_index.md b/content/docs/inference_engine/onnxruntime/_index.md
new file mode 100644
index 00000000..74774d44
--- /dev/null
+++ b/content/docs/inference_engine/onnxruntime/_index.md
@@ -0,0 +1,10 @@
+---
+weight: 401
+title: "ONNXRuntime"
+description:
+icon: menu_book
+date: 2023-09-13
+lastmod: 2023-09-13
+draft: false
+images: []
+---
diff --git a/content/docs/inference_engine/onnxruntime/infer_optim.md b/content/docs/inference_engine/onnxruntime/infer_optim.md
new file mode 100644
index 00000000..46b1ff72
--- /dev/null
+++ b/content/docs/inference_engine/onnxruntime/infer_optim.md
@@ -0,0 +1,161 @@
+---
+weight: 402
+title: "ONNXRuntime"
+description:
+icon: menu_book
+date: 2023-09-13
+lastmod: 2023-09-13
+draft: false
+images: []
+---
+
+
+
+#### 引言
+- 平时推理用的最多是ONNXRuntime,推理引擎的合适调配对推理性能有着至关重要的影响。但是有关于ONNXRuntime参数设置的资料却散落在各个地方,不能形成有效的指导意见。
+- 因此,决定在这一篇文章中来梳理一下相关的设置。
+- 以下参数都是来自`SessionOptions`中
+- 相关测试代码可以前往[AI Studio](https://aistudio.baidu.com/aistudio/projectdetail/6109918?sUid=57084&shared=1&ts=1683438418669)查看
+- 欢迎补充和指出不足之处。
+
+#### 推荐常用设置
+```python
+import onnxruntime as rt
+
+sess_options = rt.SessionOptions()
+sess_options.graph_optimization_level = rt.GraphOptimizationLevel.ORT_ENABLE_ALL
+sess_options.log_severity_level = 4
+sess_options.enable_cpu_mem_arena = False
+
+# 其他参数,采用默认即可
+```
+
+#### [`enable_cpu_mem_arena`](https://onnxruntime.ai/docs/api/python/api_summary.html#onnxruntime.SessionOptions.enable_cpu_mem_arena)
+- 作用:启用CPU上的**memory arena**。Arena可能会为将来预先申请很多内存。如果不想使用它,可以设置为`enable_cpu_mem_area=False`,默认是`True`
+- 结论:建议关闭
+ - 开启之后,占用内存会剧增(5618.3M >> 5.3M),且持续占用,不释放;推理时间只有大约13%提升
+
+- 测试环境:
+ - Python: 3.7.13
+ - ONNXRuntime: 1.14.1
+- 测试代码(来自[issue 11627](https://github.com/microsoft/onnxruntime/issues/11627),[enable_cpu_memory_area_example.zip](https://github.com/microsoft/onnxruntime/files/8772315/enable_cpu_memory_area_example.zip))
+ ```python
+ # pip install onnxruntime==1.14.1
+ # pip install memory_profiler
+
+ import numpy as np
+ import onnxruntime as ort
+ from memory_profiler import profile
+
+
+ @profile
+ def onnx_prediction(model_path, input_data):
+ ort_sess = ort.InferenceSession(model_path, sess_options=sess_options)
+ preds = ort_sess.run(output_names=["predictions"],
+ input_feed={"input_1": input_data})[0]
+ return preds
+
+
+ sess_options = ort.SessionOptions()
+ sess_options.enable_cpu_mem_arena = False
+
+ input_data = np.load('enable_cpu_memory_area_example/input.npy')
+ print(f'input_data shape: {input_data.shape}')
+ model_path = 'enable_cpu_memory_area_example/model.onnx'
+
+ onnx_prediction(model_path, input_data)
+ ```
+- Windows端 | Mac端 | Linux端 测试情况都大致相同
+
+
+ - `enable_cpu_mem_arena=True`
+ ```bash
+ (demo) PS G:> python .\test_enable_cpu_mem_arena.py
+ enable_cpu_mem_arena: True
+ input_data shape: (32, 200, 200, 1)
+ Filename: .\test_enable_cpu_mem_arena.py
+
+ Line # Mem usage Increment Occurrences Line Contents
+ =============================================================
+ 7 69.1 MiB 69.1 MiB 1 @profile
+ 8 def onnx_prediction(model_path, input_data):
+ 9 77.2 MiB 8.1 MiB 1 ort_sess = ort.InferenceSession(model_path, sess_options=sess_options)
+ 10 77.2 MiB 0.0 MiB 1 preds = ort_sess.run(output_names=["predictions"],
+ 11 5695.5 MiB 5618.3 MiB 1 input_feed={"input_1": input_data})[0]
+ 12 5695.5 MiB 0.0 MiB 1 return preds
+ ```
+ - `enable_cpu_mem_arena=False`
+ ```bash
+ (demo) PS G:> python .\test_enable_cpu_mem_arena.py
+ enable_cpu_mem_arena: False
+ input_data shape: (32, 200, 200, 1)
+ Filename: .\test_enable_cpu_mem_arena.py
+
+ Line # Mem usage Increment Occurrences Line Contents
+ =============================================================
+ 7 69.1 MiB 69.1 MiB 1 @profile
+ 8 def onnx_prediction(model_path, input_data):
+ 9 76.9 MiB 7.8 MiB 1 ort_sess = ort.InferenceSession(model_path, sess_options=sess_options)
+ 10 76.9 MiB 0.0 MiB 1 preds = ort_sess.run(output_names=["predictions"],
+ 11 82.1 MiB 5.3 MiB 1 input_feed={"input_1": input_data})[0]
+ 12 82.1 MiB 0.0 MiB 1 return preds
+ ```
+
+
+
+#### `enable_profiling`
+- 开启这个参数,在推理时,会生成一个类似`onnxruntime_profile__2023-05-07_09-02-15.json`的日志文件,包含详细的性能数据(线程、每个运算符的延迟等)。
+- 建议开启
+- 示例代码:
+ ```python
+ import onnxruntime as rt
+
+ sess_options = rt.SessionOptions()
+ sess_options.enable_profiling = True
+ ```
+
+#### `execution_mode`
+- 设置运行模型的模式,包括`rt.ExecutionMode.ORT_SEQUENTIAL`和`rt.ExecutionMode.ORT_PARALLEL`。一个序列执行,一个并行。默认是序列执行
+- **通常来说,当一个模型中有许多分支时,可以设置该参数为`ORT_PARALLEL`来达到更好的表现**
+- 当设置`sess_options.execution_mode = rt.ExecutionMode.ORT_PARALLEL`时,可以设置`sess_options.inter_op_num_threads`来控制使用线程的数量,来并行化执行(模型中各个节点之间)
+
+#### `inter_op_num_threads`
+- 设置并行化执行图(跨节点)时,使用的线程数。默认是0,交由onnxruntime自行决定。
+- 示例代码:
+ ```python
+ import onnxruntime as rt
+
+ sess_options = rt.SessionOptions()
+ sess_options.inter_op_num_threads = 2
+ ```
+
+#### `intra_op_num_threads`
+- 设置并行化执行图(内部节点)时,使用的线程数。默认是0,交由onnxruntime自行决定,一般会选择使用设备上所有的核。
+- ⚠️ 这个值并不是越大越好,具体参考[AI Studio](https://aistudio.baidu.com/aistudio/projectdetail/6109918?sUid=57084&shared=1&ts=1683438418669)中的消融实验。
+- 示例代码:
+ ```python
+ import onnxruntime as rt
+
+ sess_options = rt.SessionOptions()
+ sess_options.intra_op_num_threads = 2
+ ```
+
+#### [`graph_optimization_level`](https://github.com/microsoft/onnxruntime-openenclave/blob/openenclave-public/docs/ONNX_Runtime_Graph_Optimizations.md)
+- 运行图时,对图中算子的优化水平。默认是开启全部算子的优化。建议采用默认值即可。
+- 可选的枚举值有:`ORT_DISABLE_ALL | ORT_ENABLE_BASIC | ORT_ENABLE_EXTENDED | ORT_ENABLE_ALL`
+- 示例代码:
+ ```python
+ import onnxruntime as rt
+
+ sess_options = rt.SessionOptions()
+ sess_options.graph_optimization_level = rt.GraphOptimizationLevel.ORT_ENABLE_ALL
+ ```
+
+#### FAQ
+##### 为什么我的模型在GPU上比在CPU上还要慢?
+ - 取决于所使用的执行提供者,它可能没有完全支持模型中的所有操作。回落到CPU操作可能会导致性能速度的下降。此外,即使一个操作是由CUDA execution provider实现的,由于性能的原因,它也不一定会把操作分配/放置到CUDA EP上。要想看到ORT决定的位置,请打开verbose日志并查看控制台的输出。
+
+
+#### 参考资料
+- [ONNX Runtime Performance Tuning](https://github.com/microsoft/onnxruntime-openenclave/blob/openenclave-public/docs/ONNX_Runtime_Perf_Tuning.md)
+- [Python API](https://onnxruntime.ai/docs/api/python/api_summary.html)
diff --git a/content/docs/inference_engine/onnxruntime/onnxruntime-gpu.md b/content/docs/inference_engine/onnxruntime/onnxruntime-gpu.md
new file mode 100644
index 00000000..361053b9
--- /dev/null
+++ b/content/docs/inference_engine/onnxruntime/onnxruntime-gpu.md
@@ -0,0 +1,105 @@
+---
+weight: 403
+title: "ONNXRuntime GPU版推理"
+description:
+icon: menu_book
+date: 2023-09-13
+lastmod: 2023-09-13
+draft: false
+images: []
+---
+
+### onnxruntime-gpu版相关说明
+- 目前已知在onnxruntime-gpu上测试过的小伙伴,反映都是GPU推理速度比在CPU上慢很多。关于该问题,已经提了相关issue,具体可参见[onnxruntime issue#13198](https://github.com/microsoft/onnxruntime/issues/13198)
+
+### 有关`onnxruntime-gpu`推理慢的相关帖子
+- [Pre-allocating dynamic shaped tensor memory for ONNX runtime inference?](https://stackoverflow.com/questions/75553839/pre-allocating-dynamic-shaped-tensor-memory-for-onnx-runtime-inference)
+
+### 快速查看比较版本
+- 国外小伙伴可以基于[Google Colab](https://colab.research.google.com/gist/SWHL/673c39bf07f4cc4ddcb0e196c3e378e6/testortinfer.ipynb),国内的小伙伴可以基于百度的[AI Studio](https://aistudio.baidu.com/aistudio/projectdetail/4634684?contributionType=1&sUid=57084&shared=1&ts=1664700017761)来查看效果
+
+### 自己折腾版
+1. **onnxruntime-gpu**需要严格按照与CUDA、cuDNN版本对应来安装,具体参考[文档](https://onnxruntime.ai/docs/execution-providers/CUDA-ExecutionProvider.html#requirements),**这一步关乎后面是否可以成功调用GPU**。
+ - 以下是安装示例:
+ - 所用机器环境情况:
+ - `nvcc-smi`显示**CUDA Driver API**版本:11.7
+ - `nccc -V`显示**CUDA Runtime API**版本:11.6
+ - 以上两个版本的对应关系,可参考[博客](https://blog.csdn.net/weixin_39518984/article/details/111406728)
+ - 具体安装命令如下:
+ ```bash
+ conda install cudatoolkit=11.6.0
+ conda install cudnn=8.3.2.44
+ pip install onnxruntime-gpu==1.12.0
+ ```
+ - 验证是否可以`onnxruntime-gpu`正常调用GPU
+ 1. 验证`get_device()`是否可返回GPU
+ ```python
+ import onnxruntime as ort
+
+ print(ort.get_device())
+ # GPU
+ ```
+ 2. 如果第一步满足了,继续验证`onnxruntime-gpu`加载模型时是否可以调用GPU
+ ```python
+ import onnxruntime as ort
+
+ providers = [
+ ('CUDAExecutionProvider', {
+ 'device_id': 0,
+ 'arena_extend_strategy': 'kNextPowerOfTwo',
+ 'gpu_mem_limit': 2 * 1024 * 1024 * 1024,
+ 'cudnn_conv_algo_search': 'EXHAUSTIVE',
+ 'do_copy_in_default_stream': True,
+ }),
+ 'CPUExecutionProvider',
+ ]
+
+ # download link: https://github.com/openvinotoolkit/openvino/files/9355419/super_resolution.zip
+ model_path = 'super_resolution.onnx'
+ session = ort.InferenceSession(model_path, providers=providers)
+
+ print(session.get_providers())
+ # 如果输出中含有CUDAExecutionProvider,则证明可以正常调用GPU
+ # ['CUDAExecutionProvider', 'CPUExecutionProvider']
+ ```
+2. 更改[`config.yaml`](https://github.com/RapidAI/RapidOCR/blob/main/python/rapidocr_onnxruntime/config.yaml)中对应部分的参数即可,详细参数介绍参见[官方文档](https://onnxruntime.ai/docs/execution-providers/CUDA-ExecutionProvider.html)。
+ ```yaml
+ use_cuda: true
+ CUDAExecutionProvider:
+ device_id: 0
+ arena_extend_strategy: kNextPowerOfTwo
+ gpu_mem_limit: 2 * 1024 * 1024 * 1024
+ cudnn_conv_algo_search: EXHAUSTIVE
+ do_copy_in_default_stream: true
+ ```
+
+3. 推理情况
+ 1. 下载基准测试数据集(`test_images_benchmark`),放到`tests/benchmark`目录下。
+ - [百度网盘](https://pan.baidu.com/s/1R4gYtJt2G3ypGkLWGwUCKg?pwd=ceuo) | [Google Drive](https://drive.google.com/drive/folders/1IIOCcUXdWa43Tfpsiy6UQJmPsZLnmgFh?usp=sharing)
+ - 最终目录结构如下:
+ ```text
+ tests/benchmark/
+ ├── benchmark.py
+ ├── config_gpu.yaml
+ ├── config.yaml
+ └── test_images_benchmark
+ ```
+ 2. 运行以下代码(`python`目录下运行):
+ ```shell
+ # CPU
+ python tests/benchmark/benchmark.py --yaml_path config.yaml
+
+ # GPU
+ python tests/benchmark/benchmark.py --yaml_path config_gpu.yaml
+ ```
+ 3. 运行相关信息汇总:(以下仅为个人测试情况,具体情况请自行测试)
+ - 环境
+ |测试者|设备|OS|CPU|GPU|onnxruntime-gpu|
+ |:--|:--|:--|:--|:--|:--|
+ |[1][zhsunlight](https://github.com/zhsunlight)|宏碁(Acer) 暗影骑士·威N50-N93游戏台式机|Windows|十代i5-10400F 16G 512G SSD|NVIDIA GeForce GTX 1660Super 6G|1.11.0|
+ |[2][SWHL](https://github.com/SWHL)|服务器|Linux|AMD R9 5950X|NVIDIA GeForce RTX 3090|1.12.1|
+ - 耗时
+ |对应上面序号|CPU总耗时(s)|CPU平均耗时(s/img)|GPU总耗时(s)|GPU平均耗时(s/img)||
+ |:---:|:---:|:---:|:---:|:---:|:---:|
+ |[1]|296.8841|1.18282|646.14667|2.57429|
+ |[2]|149.35427|0.50504|250.81760|0.99927|
\ No newline at end of file
diff --git a/content/docs/inference_engine/openvino/_index.md b/content/docs/inference_engine/openvino/_index.md
new file mode 100644
index 00000000..b45d4984
--- /dev/null
+++ b/content/docs/inference_engine/openvino/_index.md
@@ -0,0 +1,10 @@
+---
+weight: 402
+title: "OpenVINO"
+description:
+icon: menu_book
+date: 2023-09-13
+lastmod: 2023-09-13
+draft: false
+images: []
+---
diff --git a/content/docs/inference_engine/openvino/infer-gpu.md b/content/docs/inference_engine/openvino/infer-gpu.md
new file mode 100644
index 00000000..e122b87c
--- /dev/null
+++ b/content/docs/inference_engine/openvino/infer-gpu.md
@@ -0,0 +1,13 @@
+---
+weight: 403
+title: "OpenVINO GPU推理"
+description:
+icon: menu_book
+date: 2023-09-13
+lastmod: 2023-09-13
+draft: false
+images: []
+---
+
+- 官方参考文档:[docs](https://docs.openvino.ai/latest/api/ie_python_api/_autosummary/openvino.runtime.Core.html?highlight=compile_model#openvino.runtime.Core.compile_model)
+- 考虑到openvino只能使用自家显卡推理,通用性不高,这里暂不作相关配置说明。
\ No newline at end of file
diff --git a/content/docs/inference_engine/openvino/infer.md b/content/docs/inference_engine/openvino/infer.md
new file mode 100644
index 00000000..cc632269
--- /dev/null
+++ b/content/docs/inference_engine/openvino/infer.md
@@ -0,0 +1,91 @@
+---
+weight: 403
+title: "OpenVINO推理"
+description:
+icon: menu_book
+date: 2023-09-13
+lastmod: 2023-09-13
+draft: false
+images: []
+---
+
+- ⚠️ 基于目前`openvino==2022.3.0`版,存在申请内存不释放的问题,这也就意味着当推理图像很大时,推理完之后,内存会一直占用。详情可参见[issue11939](https://github.com/openvinotoolkit/openvino/issues/11939)
+
+### 安装
+```bash
+$ pip install openvino
+
+# 里面含有mo
+$ pip install openvino-dev
+```
+
+### 模型问题
+- 因为OpenVINO可以直接推理ONNX模型,故这里暂时不作转换,直接推理之前ONNX模型即可
+- 这里仍然给出转换的代码,用作参考:
+ ```bash
+ mo --input_model models/ch_PP-OCRv2_det_infer.onnx --output_dir models/IR/
+
+ mo --input_model models/ch_PP-OCRv2_det_infer.onnx \
+ --output_dir models/IR/static \
+ --input_shape "[1,3,12128,800]"
+ ```
+
+### 关于OpenVINO
+- OpenVINO可以直接推理IR、ONNX和PaddlePaddle模型,具体如下(图来源:[link](https://docs.openvino.ai/latest/openvino_docs_OV_UG_OV_Runtime_User_Guide.html#doxid-openvino-docs-o-v-u-g-o-v-runtime-user-guide)):
+
+
+

+
+
+- 和ONNXRuntime同时推理同一个ONNX模型,OpenVINO推理速度更快
+- 但是从对比来看,OpenVINO占用内存更大,其原因是拿空间换的时间
+ - 当指定`input_shape`在一个区间范围时,推理时内存占用会减少一些
+ - 示例命令:
+ ```bash
+ mo --input_model models/ch_PP-OCRv2_det_infer.onnx \
+ --output_dir models/IR/static \
+ --input_shape "[1,3,960:1200,800]"
+ ```
+
+### OpenVINO与ONNXRuntime性能对比
+- 推理设备:`Windows 64位 Intel(R) Core(TM) i5-4210M CPU @ 2.60GHz 2.59 GHz`
+- [测试图像宽高](https://drive.google.com/file/d/1iJcGvOVIdUlyOS52bBdvO8uzx8QORo5M/view?usp=sharing): `12119x810`
+
+| 测试模型 | 推理框架 | 占用内存(3次平均) | 推理时间(3次平均) |
+| ------------------------------------ | -------------------- | ----------------- | ----------------- |
+| `ch_PP-OCRv2_det_infer.onnx` | `ONNXRuntime=1.10.0` | 0.8G | 5.354s |
+| `ch_PP-OCRv2_det_infer.onnx` | `openvino=2022.1.0` | 3.225G | 2.53s |
+| `ch_PP-OCRv2_det_infer.xml` FP32 动态图 | `openvino=2022.1.0` | 3.175G | 2.0455s |
+
+
+### OpenVINO与ONNXRuntime推理代码写法对比
+NOTE: 以`ch_ppocr_mobile_v2_det`中推理代码为例子
+- ONNXRuntime
+ ```python
+ import onnxruntime
+
+ # 声明
+ sess_opt = onnxruntime.SessionOptions()
+ sess_opt.log_severity_level = 4
+ sess_opt.enable_cpu_mem_arena = False
+ session = onnxruntime.InferenceSession(det_model_path, sess_opt)
+ input_name = session.get_inputs()[0].name
+ output_name = session.get_outputs()[0].name
+
+ # 推理
+ preds = session.run([output_name], {input_name: img})
+ ```
+- OpenVINO
+ ```python
+ from openvino.runtime import Core
+
+ # 初始化
+ ie = Core()
+ model_onnx = ie.read_model(det_model_path)
+ compile_model = ie.compile_model(model=model_onnx, device_name='CPU')
+ vino_session = compile_model.create_infer_request()
+
+ # 推理
+ vino_session.infer(inputs=[img])
+ vino_preds = vino_session.get_output_tensor().data
+ ```