You signed in with another tab or window. Reload to refresh your session.You signed out in another tab or window. Reload to refresh your session.You switched accounts on another tab or window. Reload to refresh your session.Dismiss alert
DEFINE_string(model_dir, "", "Directory of the inference model. "
"It constains deploy.yaml and infer models");
DEFINE_string(img_path, "", "Path of the test image.");
DEFINE_bool(use_cpu, false, "Wether use CPU. Default: use GPU.");
DEFINE_bool(use_trt, false, "Wether enable TensorRT when use GPU. Defualt: false.");
DEFINE_bool(use_mkldnn, false, "Wether enable MKLDNN when use CPU. Defualt: false.");
DEFINE_string(save_dir, "", "Directory of the output image.");
int main(int argc, char *argv[]) {
google::ParseCommandLineFlags(&argc, &argv, true);
if (FLAGS_model_dir == "") {
LOG(FATAL) << "The model_dir should not be empty.";
}
// Prepare data
cv::Mat img = read_process_image(yaml_config.is_normalize);
int rows = img.rows;
int cols = img.cols;
int chs = img.channels();
std::vector input_data(1 * 3 * 1080 *1920, 0.0f);
hwc_img_2_chw_data(img, input_data.data());
// Create predictor
auto predictor = create_predictor(yaml_config);
// Set input
auto input_names = predictor->GetInputNames();
auto input_t = predictor->GetInputHandle(input_names[0]);
std::vector input_shape = {1, 3, 1080, 1920};
input_t->Reshape(input_shape);
input_t->CopyFromCpu(input_data.data());
// Run
clock_t start,end;
start=clock();
predictor->Run();
end=clock();
cout << "The run time is:" << (double)(end-start)/CLOCKS_PER_SEC << "s" << endl;
// Get output
auto output_names = predictor->GetOutputNames();
auto output_t = predictor->GetOutputHandle(output_names[0]);
std::vector output_shape = output_t->shape(); // n * h * w
int out_num = std::accumulate(output_shape.begin(), output_shape.end(), 1,
std::multiplies());
std::vector<int64_t> out_data(out_num);
output_t->CopyToCpu(out_data.data());
// Get pseudo image
std::vector<uint8_t> out_data_u8(out_num);
for (int i = 0; i < out_num; i++) {
out_data_u8[i] = static_cast<uint8_t>(out_data[i]);
}
cv::Mat out_gray_img(output_shape[1], output_shape[2], CV_8UC1, out_data_u8.data());
cv::Mat out_eq_img;
cv::equalizeHist(out_gray_img, out_eq_img);
cv::imwrite("out_img.jpg", out_eq_img);
这是我参考的例程,
这是我的推理时间:
以下是全部代码:
#include
#include
#include
#include
#include
#include <time.h>
#include <gflags/gflags.h>
#include <glog/logging.h>
using namespace std;
#include "paddle/include/paddle_inference_api.h"
#include "yaml-cpp/yaml.h"
#include "opencv2/core.hpp"
#include "opencv2/imgproc.hpp"
#include "opencv2/highgui.hpp"
DEFINE_string(model_dir, "", "Directory of the inference model. "
"It constains deploy.yaml and infer models");
DEFINE_string(img_path, "", "Path of the test image.");
DEFINE_bool(use_cpu, false, "Wether use CPU. Default: use GPU.");
DEFINE_bool(use_trt, false, "Wether enable TensorRT when use GPU. Defualt: false.");
DEFINE_bool(use_mkldnn, false, "Wether enable MKLDNN when use CPU. Defualt: false.");
DEFINE_string(save_dir, "", "Directory of the output image.");
typedef struct YamlConfig {
std::string model_file;
std::string params_file;
bool is_normalize;
}YamlConfig;
YamlConfig load_yaml(const std::string& yaml_path) {
YAML::Node node = YAML::LoadFile(yaml_path);
std::string model_file = node["Deploy"]["model"].asstd::string();
std::string params_file = node["Deploy"]["params"].asstd::string();
bool is_normalize = false;
if (node["Deploy"]["transforms"] &&
node["Deploy"]["transforms"][0]["type"].asstd::string() == "Normalize") {
is_normalize = true;
}
YamlConfig yaml_config = {model_file, params_file, is_normalize};
return yaml_config;
}
std::shared_ptr<paddle_infer::Predictor> create_predictor(const YamlConfig& yaml_config) {
std::string& model_dir = FLAGS_model_dir;
paddle_infer::Config infer_config;
infer_config.SetModel(model_dir + "/" + yaml_config.model_file,
model_dir + "/" + yaml_config.params_file);
infer_config.EnableMemoryOptim();
if (FLAGS_use_cpu) {
LOG(INFO) << "Use CPU";
if (FLAGS_use_mkldnn) {
// TODO(jc): fix the bug
//infer_config.EnableMKLDNN();
infer_config.SetCpuMathLibraryNumThreads(5);
}
} else {
LOG(INFO) << "Use GPU";
infer_config.EnableUseGpu(500, 0);
if (FLAGS_use_trt) {
infer_config.EnableTensorRtEngine(1 << 30, 1, 1,
paddle_infer::PrecisionType::kFloat32, false, false);
}
}
auto predictor = paddle_infer::CreatePredictor(infer_config);
return predictor;
}
void hwc_img_2_chw_data(const cv::Mat& hwc_img, float* data) {
int rows = hwc_img.rows;
int cols = hwc_img.cols;
int chs = hwc_img.channels();
for (int i = 0; i < chs; ++i) {
cv::extractChannel(hwc_img, cv::Mat(rows, cols, CV_32FC1, data + i * rows * cols), i);
}
}
cv::Mat read_process_image(bool is_normalize) {
cv::Mat img = cv::imread(FLAGS_img_path, cv::IMREAD_COLOR);
cv::cvtColor(img, img, cv::COLOR_BGR2RGB);
if (is_normalize) {
img.convertTo(img, CV_32F, 1.0 / 255, 0);
img = (img - 0.5) / 0.5;
}
return img;
}
int main(int argc, char *argv[]) {
google::ParseCommandLineFlags(&argc, &argv, true);
if (FLAGS_model_dir == "") {
LOG(FATAL) << "The model_dir should not be empty.";
}
// Load yaml
std::string yaml_path = FLAGS_model_dir + "/deploy.yaml";
YamlConfig yaml_config = load_yaml(yaml_path);
// Prepare data
cv::Mat img = read_process_image(yaml_config.is_normalize);
int rows = img.rows;
int cols = img.cols;
int chs = img.channels();
std::vector input_data(1 * 3 * 1080 *1920, 0.0f);
hwc_img_2_chw_data(img, input_data.data());
// Create predictor
auto predictor = create_predictor(yaml_config);
// Set input
auto input_names = predictor->GetInputNames();
auto input_t = predictor->GetInputHandle(input_names[0]);
std::vector input_shape = {1, 3, 1080, 1920};
input_t->Reshape(input_shape);
input_t->CopyFromCpu(input_data.data());
// Run
clock_t start,end;
start=clock();
predictor->Run();
end=clock();
cout << "The run time is:" << (double)(end-start)/CLOCKS_PER_SEC << "s" << endl;
// Get output
auto output_names = predictor->GetOutputNames();
auto output_t = predictor->GetOutputHandle(output_names[0]);
std::vector output_shape = output_t->shape(); // n * h * w
int out_num = std::accumulate(output_shape.begin(), output_shape.end(), 1,
std::multiplies());
std::vector<int64_t> out_data(out_num);
output_t->CopyToCpu(out_data.data());
// Get pseudo image
std::vector<uint8_t> out_data_u8(out_num);
for (int i = 0; i < out_num; i++) {
out_data_u8[i] = static_cast<uint8_t>(out_data[i]);
}
cv::Mat out_gray_img(output_shape[1], output_shape[2], CV_8UC1, out_data_u8.data());
cv::Mat out_eq_img;
cv::equalizeHist(out_gray_img, out_eq_img);
cv::imwrite("out_img.jpg", out_eq_img);
LOG(INFO) << "Finish";

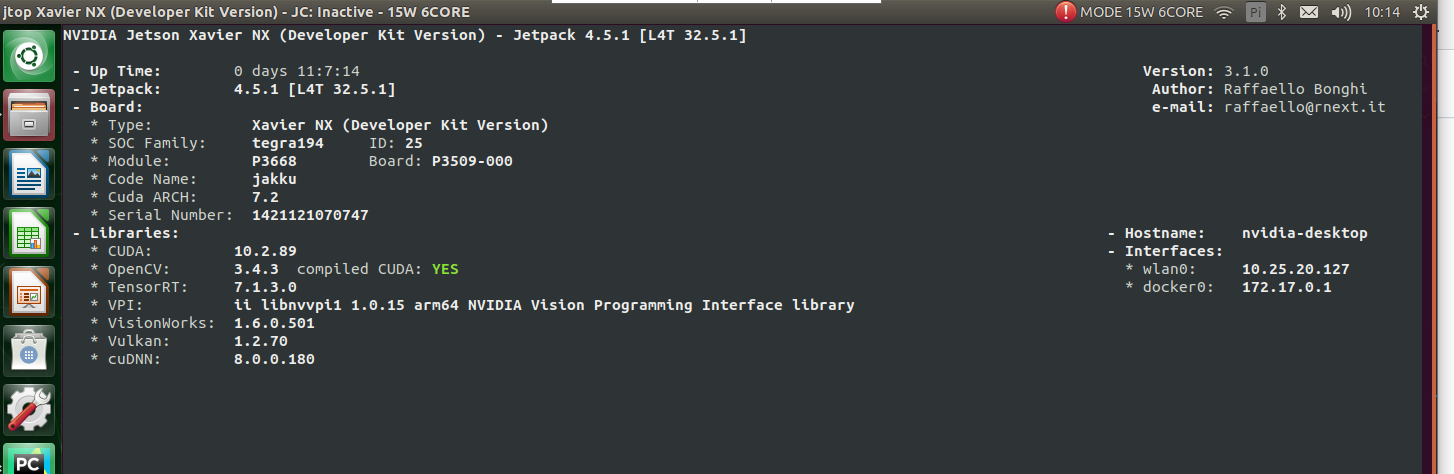
}
paddle 版本2.1.2:
nx 环境:
输入图像大小为1080*1920。
The text was updated successfully, but these errors were encountered: